In the world of data analytics, reaching the stage where predictive insights guide your business decisions is akin to making it to the playoffs in sports. It’s an achievement that many organizations strive for but only a few manage to realize. To get to this coveted stage, teams need to go through rigorous training and preparation, which in the context of analytics means mastering descriptive and diagnostic analytics first.
Building the Foundation: Descriptive and Diagnostic Analytics
Before a team can compete for the championship, they need to build a strong foundation. In analytics, this foundational work is done through descriptive and diagnostic analytics.
- Descriptive Analytics: This is where you analyze historical data to understand what has happened. It’s like reviewing past games to see how the team performed, identifying strengths, and areas for improvement.
- Example: A retail company uses descriptive analytics to summarize its sales data from the past year, identifying peak sales periods and best-selling products. This helps in understanding overall performance and trends.
- Diagnostic Analytics: Once you know what happened, the next step is to understand why it happened. Diagnostic analytics digs deeper into the data to uncover the causes behind the trends and patterns.
- Example: The same retail company applies diagnostic analytics to figure out why certain products sold better during specific periods. This could involve analyzing factors like marketing campaigns, customer demographics, and economic conditions.
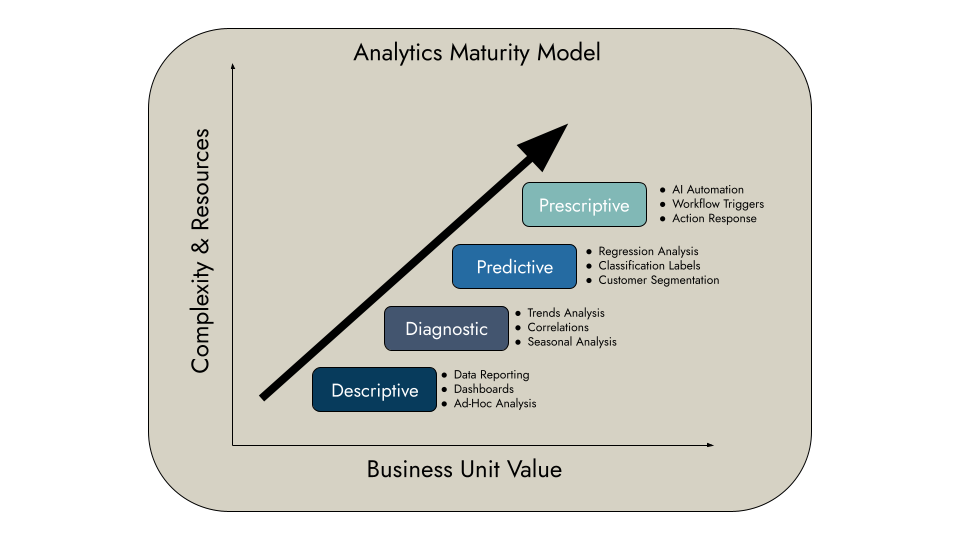
These two forms of analytics are essential to prepare an organization for predictive analytics. They are like the regular season games that prepare the team for the intense competition of the playoffs.
Stepping Up: Embracing Predictive Analytics
With a solid foundation, it’s time to step up to predictive analytics—the playoffs of data analytics. Predictive analytics uses historical data to forecast future outcomes, helping organizations make data-driven decisions with confidence.
- Supervised Learning: This method uses labeled datasets to train algorithms that can predict future outcomes based on past data. It’s like a team studying their playbook and past games to predict and plan for the opponent’s moves.
- Example: A bank uses supervised learning to predict which customers are likely to default on their loans. By analyzing past data on customer behavior and loan defaults, they can proactively manage risk and take preventive measures.
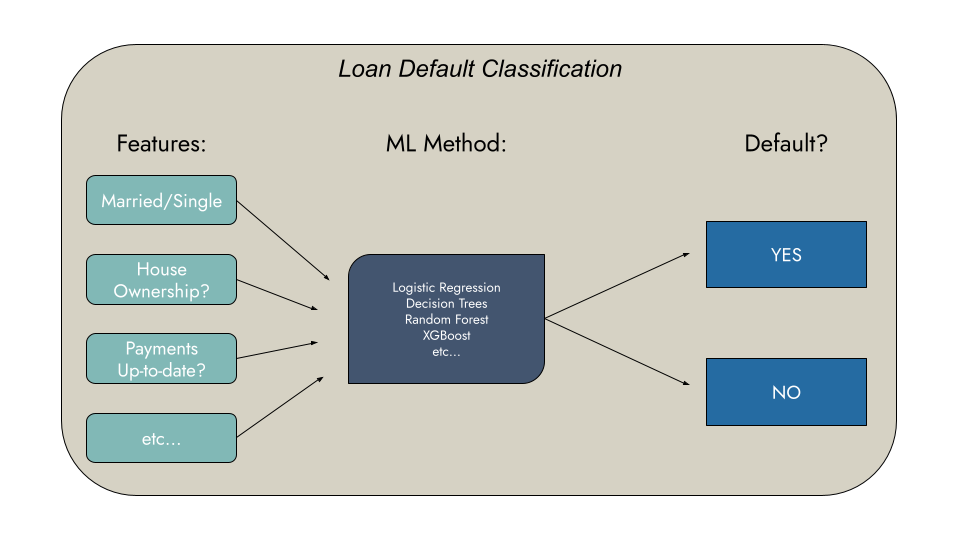
- Unsupervised Learning: Unlike supervised learning, unsupervised learning doesn’t rely on labeled data. Instead, it finds patterns and insights from data without predefined outcomes. It’s similar to a coach analyzing player behaviors and forming strategies without having a clear playbook.
- Example: A marketing team uses unsupervised learning to segment their customers into distinct groups based on purchasing behavior. This helps in tailoring marketing strategies to different customer segments for better engagement and retention.
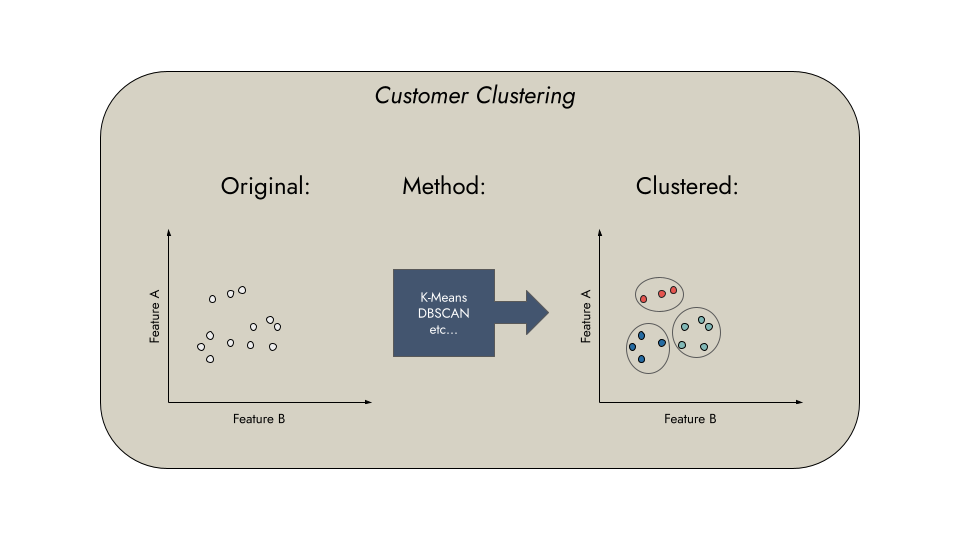
Ensuring Success: Data Quality and Infrastructure
Just as a sports team needs the right equipment and training facilities, successful predictive analytics relies on high-quality data and robust infrastructure.
- Data Quality: Ensuring your data is accurate, clean, and relevant is crucial. Garbage data leads to poor predictions, just as inadequate training leads to poor performance in games.
- Example: A sales forecasting model requires clean data. If the data includes incorrect entries or mismatches, it can lead to inaccurate predictions, much like a team relying on flawed strategies.
- Infrastructure: Having the right tools and resources is essential for preparing and analyzing data. This is akin to having the best training facilities and equipment for athletes.
- Example: Organizations might use cloud platforms like AWS or Google Cloud for scalable data storage and processing, along with tools like Tableau or Power BI for data analysis.
The following tools enable businesses of various sizes to achieve their data milestones. This list is not ranked in any hierarchy or preference—it simply includes tools that came to mind while writing this. Many other excellent tools exist and can be considered based on your specific needs.
- Cloud Data Warehouses for Storage and Computation:
- Examples: Snowflake, Google Cloud Platform (GCP), Amazon Web Services (AWS), Microsoft Azure, IBM Cloud
- Data Wrangling Technologies & IDEs for Data Analysts, Data Engineers, and Data Scientists:
- Examples: dbt, Dataiku, Alteryx, Hex, Jupyter Notebooks, Visual Studio Code, Informatica
- Data Visualization Tools for Displaying Results to Stakeholders:
- Examples: Tableau, Power BI, Qlik, Looker
Depending on the project and available licenses at your organization or the partners you are working with, different tools might be a good fit. A good spot for research around best tools can start with Gartner’s Magic Quadrants, they have specific research around leaders in the space, such as Gartner’s Magic Quadrant for Analytics and Business Intelligence Platforms.
Incorporating Expertise: Subject Matter Experts (SMEs)
To make it to the playoffs and succeed, teams need not only great players but also expert coaches. In analytics, Subject Matter Experts (SMEs) play this crucial role. A model with 75% accuracy might be transformative for a marketing campaign in an e-commerce setting but could be dangerously inadequate for a cancer-predicting medical device.
- Role of SMEs: SMEs provide the necessary domain knowledge and insights that ensure the analytics models are relevant and actionable. They help interpret the data in the context of the business and guide the predictive models to align with strategic goals.
- Example: In healthcare, SMEs can guide the development of predictive models for patient care by providing insights into medical practices and patient needs.
Staying Competitive: Continuous Monitoring and Improvement
Reaching the playoffs is just the beginning. To stay competitive, teams need continuous practice and adaptation. Similarly, predictive models require ongoing monitoring and refinement. Some might consider it like running… Not the most enjoyable (personal opinion) but necessary to stay in shape.
- Performance Monitoring: Regularly tracking the accuracy and reliability of predictive models is essential. This is similar to reviewing game performance to make tactical adjustments.
- Example: A retail company continually monitors its sales prediction model to ensure it adapts to new market trends and customer behaviors.
- Bias Detection and Mitigation: It’s important to assess models for biases and make necessary adjustments. Just as teams work on strategies to play fairly and inclusively, analytics teams need to ensure their models do not perpetuate biases.
- Example: A recruitment algorithm should be regularly evaluated to ensure it does not favor candidates based on gender or ethnicity.
Embracing the Journey: From Descriptive to Predictive Analytics
Getting to the playoffs is a journey of growth and learning. Similarly, moving from descriptive and diagnostic analytics to predictive analytics is a transformative journey for organizations. Each step builds on the previous one, leading to more sophisticated and impactful data-driven decision-making.
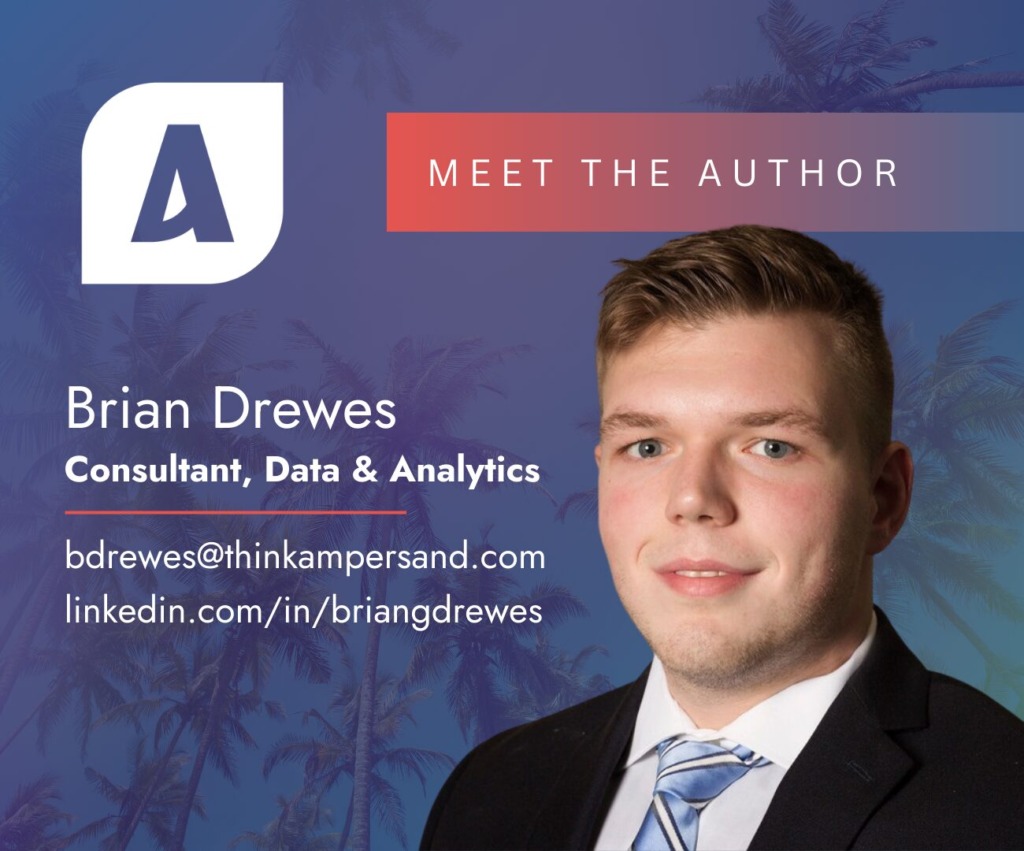
Brian is a versatile Data & Analytics Consultant with expertise in analytics, machine learning, sales strategy, and business development, excelling in integrating customer insights, data science, business intelligence, and technology enablement. Known for his team-centric and empathetic approach, he effectively drives both strategic initiatives and technical projects, empowering individuals to maximize their potential.